In this third article, we transition from implementing the key processes of leading AI-enterprises to fruitfully building your own AI projects. Our objective for this series is to address more of the symptoms of an academic AI strategy and demonstrate how to build practical AI projects that augment your enterprise strategy. We will discuss project workflows, selecting projects, avoiding common pitfalls, effectively organizing teams, spotting automatable tasks, optimizing processes, and performing due diligence. Accordingly, we provide a roadmap that will empower you to strategically develop exciting and valuable projects for your enterprise today.
Determining if Your Enterprise is Ready to Adopt AI
A challenging question that many organizations struggle with is understanding when their business is ready to start using AI. This is a significant question to answer but a more useful one is determining if your enterprise could benefit from adopting AI into your business model. The best approach to this question is to develop a basic knowledge of AI technology by learning what it can and cannot do, and then decide if these new capabilities could help drive business value or solve pain points in your organization. Once you develop an intuition of what AI is, then you could start brainstorming potentially feasible and valuable pilot projects that can be executed using in-house expertise or a partner.
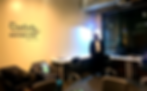
AI Systems vs Data Science Workflows
To begin the process of developing fruitful pilot projects, it is essential to learn the workflows for building AI systems and data science projects. The main drivers of value for AI systems and data science projects are machine learning and data. Machine learning is the task of automatically mapping some input A to output B that usually results in a running AI system such as a website, mobile app, or autonomous device produced by an AI engineer or team. Building a running AI system involves key steps such as collecting data, iteratively training a machine learning algorithm, and deploying the model as an intelligent software system or autonomous device. More complex AI projects may require additional steps depending on the application, but this general workflow is applicable for most AI solutions.
Another important tool in AI that helps computers behave intelligently is data science. Data science is the science of analyzing data to extract a set of insights to help make business decisions by a data scientist or team. The key steps of a data science project include collecting data, iteratively analyzing data, and preparing a slide deck or presentation with a set of actionable insights that suggest hypotheses or actions. Many organizations struggle with understanding the difference between AI systems and data science workflows. However, the main takeaway is to understand that the output of an AI system will map some input to an output to automate a given task similar to a smart speaker. Whereas the output of a data science project will enable you to gain actionable insights to improve decision making or optimize a given process.
Finding The Right Use Cases
AI is now more accessible than ever before, but another challenge that enterprises face during adoption is identifying the right use cases. This is a challenging problem because many applications of AI cannot be implemented as a SaaS solution that you purchase and install, and then instantly become AI-enabled. In fact, AI requires a strategic framework to ensure you select projects that are both feasible and valuable for your use case. Also, it requires architecting your organization to achieve what modern AI capabilities allow you to do well. Notably, the impact of AI on many industry verticals is strategic rather than tactical. Therefore, it is imperative for enterprises to address this challenge strategically because AI has the ability to transform the core of how many companies operate.
To help enterprises improve their ability to identify the right use cases, we will provide a strategic framework to help verify if an idea may be fruitful or not. We recommend starting small by identifying pilot projects that can help you gain momentum instead of executing larger projects that have a lesser chance of initial success. Importantly, the pilot project doesn’t have to be the most lucrative idea but it should be completed successfully, and help you on your journey with executing more valuable ideas.
A strategic framework that can improve your adoption of AI is performing due diligence. This is the process of pairing AI talent and subject matters experts to perform both technical and business diligence before committing to executing projects. Performing technical diligence will enable AI teams to validate if an idea can achieve the desired performance, confirm how much data is required, set engineering timelines, and consider ethical questions during development. Business diligence enables domain experts to collaborate with AI experts to find valuable use cases for your business. Examples of potential use cases that could drive value include projects that lower costs, improve efficiencies, and increase revenues. Additionally, conducting business diligence can empower enterprises to improve their existing business operations, and also launch new businesses or products.
Common Pitfalls to Avoid When Identifying Use Cases
In addition to applying the due diligence framework, we will highlight some common pitfalls to avoid on your journey. The first suggestion we recommend is for organizations to have a realistic view about AI capabilities and limitations. AI is already transforming many industries but it is important to incorporate technical, business, and ethical diligence while considering potential use cases. A second suggestion we recommend is to think about acquiring data strategically by combining AI talent and domain experts early in the process. If you can get engineering and business talent to collaborate earlier in the process to acquire valuable data, then finding feasible and valuable projects will be more effective.
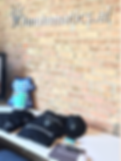
Our third suggestion is for teams to implement modern processes to work more effectively as AI continues to evolve into a systematic engineering discipline. Some new ideas include planning for AI projects to be an iterative process where development may require multiple attempts to succeed. This also includes the use of newer innovative agile development ideas such as one-day sprints instead of traditional two-week sprints. Innovating on the processes to figure out how to get cross-functional teams to work together more systematically and effectively will transform how machine learning systems are built in the future.
A fourth suggestion to reinforce the previous idea of newer development processes is to explore better ways of planning for AI projects. As AI evolves into a systematic engineering discipline, traditional planning processes will also need to change. This task will require forming cross-functional teams with knowledgeable AI experts that can establish timeline estimates, milestones, and performance metrics appropriate for AI projects. The fifth suggestion we recommend is to start small if you don’t have big data or a large team of AI experts to execute on an idea. Many organizations falsely believe that they need vast amounts of data or superstar AI talent to get started. This is true in some larger and more complex use cases. However, some innovative ideas to address this concern include applying machine learning techniques that require less data such as few-shot learning, data generation, data augmentation, transfer learning, and self-supervised learning. Relevantly, the rise of small data in AI will allow this disruptive technology to break into many new industries that do not have big data assets to generate new value. Also, investing in training, acquiring in-house AI talent, or working with a partner can help enterprises gain momentum faster.
Working in Cross-functional Teams
An important trend that we see across leading AI enterprises is the pairing of AI and subject matter expertise to form cross-functional teams. AI experts can help your team quickly understand the capabilities and limitations of AI technology. Whereas domain experts can also help you quickly identify what is most valuable to your business.
Therefore, the best approach to selecting feasible and valuable projects is to bring both teams together to collaboratively explore fruitful use cases. After forming a cross-functional team, you will be better positioned to deeply evaluate whether an idea is something AI can do, as well as know how valuable it is to the business. Ideally, the cross-functional team will brainstorm at least six potential projects to explore, and then narrow it down to one or two pilot projects to invest in. Lastly, due to the scarcity of AI talent, it is very common to collaborate with a partner on executing ideal projects for your specific business problem.
Automating Tasks and Not Jobs
Now that you have seen how to combine AI and domain knowledge to select worthwhile projects, next we will address why enterprises should think of automating tasks instead of jobs. This is an important societal issue to address across every industry sector because the set of tasks that are now automatable in certain job functions is much higher today. As a result, automation will have an accelerated impact on certain jobs worldwide.
If a job function comprises of many tasks that are suitable for automation, then the risk of that job function being displaced will be much higher. Job functions that comprise of monotonous and repetitive work are at higher risk of automation. These include food preparation, construction, cleaning, driving, and agricultural labor. Interestingly, recent surveys have estimated that more jobs will be created than displaced by 2030. Therefore, some useful solutions to reduce the impact of AI automation include preparing for new roles that will be created by AI in the future, lifelong learning, an income safety net, and incentivized learning.
Although some routine and repetitious job functions are at risk, many other roles that require more social interaction will be impossible to automate, and will instead be augmented with AI. We recommend that while brainstorming potential AI applications, it is useful to think about automating common tasks that could be performed in under a second rather than entire jobs. A good way to approach this issue is to review the tasks that comprise a job and select the tasks that are suitable for automation and would allow you to create value in other areas. Likewise, working in cross-functional teams and considering ethics is an optimal method to find the right use cases.
Questions to Consider While Brainstorming Ideas
While thinking about automating tasks, there are some key questions that teams can ask that could lead to valuable ideas. A fundamental question to ask when performing business diligence is to determine what are the main drivers of business value. Once you have determined what drives value, then you may be able to find data science or AI solutions to augment your corporate strategy.
Another important question to consider is finding the pain points in your business. Understanding the pain points presents a huge opportunity to use AI technology to help solve these issues. Lastly, another useful question to ask that will position you for long term success is whether your organization could be more effective and valuable if it were great at AI.
By asking these important questions during the brainstorming process, you will have a much better idea of fruitful projects that will improve how you create value and compete in the future. Finally, you will gain a better intuition of how to architect your company to take full advantage of modern AI capabilities to become a leader in your industry.

AI Services and Transformative Experiences
What We Do
funstematics.ai is an AI services and research company that provides AI-powered solutions and transformative experiences for enterprises.
We Create Value in Two Key Ways:
1. AI services and products that help you produce running AI systems that automate tasks, and data science projects that optimize business processes and extract actionable insights for decisions.
2. Secondly, our AI transformation process for enterprises provide a roadmap to evolve into an AI-first company by developing pilots to gain momentum faster, creating a modern AI and data strategy, performing training, building in-house capabilities, aligning stakeholders, and considering ethics.
We are unique in that we provide all of the key AI application areas that a vertical company would provide such as computer vision, natural language processing, speech recognition, reinforcement learning, generative modeling, network science, GIS, and recommender systems for a variety of tasks or problems. Furthermore, our research is focused on creating value by developing brand new applications of AI using multimodal systems.
Technology Services
funstematics.ai has strategic partnerships with key leading and emerging AI, open-source, commercial SaaS, cloud, data management, software, and systems engineering vendors around the world.
In addition to AI systems and research, we also provide the following services:
Data Science
Business Intelligence
Data Visualization & Exploratory Data Analysis
Big Data, Cloud, IoT, Edge, HPC, Data Warehouse
Blockchain Development
Testing as a Service, DevOps Development, Software & Systems Engineering
Microservices, Containers, Orchestration
Scientific Research & Engineering
GIS, Spatial Analysis, Remote Sensing
Custom Web Application Development, Mobile Apps - iOS, Android
Data Collection & Surveying, Strategic Data Acquisition
Project Management, Scrum & Lean Six Sigma
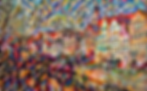
Get Started on this Fun and Exciting Journey into the AI Era Today
If you are interested in learning how you can apply this transformative technology to build valuable applications and lead your enterprise into the AI era today, contact our sales team to get started. www.funstematics.ai
Conclusion
In this article, we discussed how to strategically select and build valuable AI systems and data science projects. Specifically, we explained the workflows that AI engineers and data scientists use to build solutions. We also provided a systematic framework for selecting potential AI use cases. Then we discussed how to address some of the common AI pitfalls that teams face while developing projects. In addition, we explained the purpose and value of forming cross-functional teams when adopting AI. Furthermore, we discussed the important issue of job automation and how to think about automating tasks instead of jobs. The next articles will provide more useful solutions to help enterprises achieve long-term success using this transformative technology.
Is there a specific challenge or interesting topic you would like us to cover in a future article? Then leave a comment below.
Interested in starting your AI project, or identifying new valuable and feasible AI opportunities for your business? Let's talk!
